This project took some time to get working, what it involved was getting the GPU acceleration for the two major frameworks
go to this link: to install drivers
then install cockpit
sudo dnf install cockpit cockpit-podman podmancockpit systemctl enable --now cockpit.socket
Open a web browser and go to http://localhost:9090
and go to command prompt in cockpit, make sure NVIDIA container toolkit is installed
podman run --device nvidia.com/gpu=all -p 8888:8889 -it docker.io/pytorch/pytorch:latest
For Pytorch
podman run --device nvidia.com/gpu=all -p 8888:8889 -it nvcr.io/nvidia/tensorflow:24.01-tf2-py3
#for tensorflow for GPU inside the pod, install jupyter
pip install jupyter
#to get jupyter running, Make sure to make a firewall port 8888 and 8889 in the cockpit
jupyter notebook --ip=0.0.0.0 --port=8889 --no-browser --allow-root
#connect to jupyter through link 8888 if that doesn't work (it's finecky), and put in your token and log in. To test tensorflow #TORCH:
import torch
def check_gpu():
if torch.cuda.is_available():
print("CUDA is available. Number of GPUs available:", torch.cuda.device_count())
print("Details of each GPU:")
for i in range(torch.cuda.device_count()):
print(f"GPU {i}: {torch.cuda.get_device_name(i)}")
print(f" Memory Allocated: {torch.cuda.memory_allocated(i)} bytes")
print(f" Memory Cached: {torch.cuda.memory_reserved(i)} bytes")
else:
print("CUDA is not available. No GPU detected.")
check_gpu()
#TENSORFLOW:
import tensorflow as tf
def check_tf_gpu():
if tf.config.list_physical_devices('GPU'):
print("TensorFlow is using the GPU(s).")
gpus = tf.config.list_physical_devices('GPU')
for gpu in gpus:
print(f"GPU: {gpu.name}")
else:
print("TensorFlow is not using the GPU.")
check_tf_gpu()
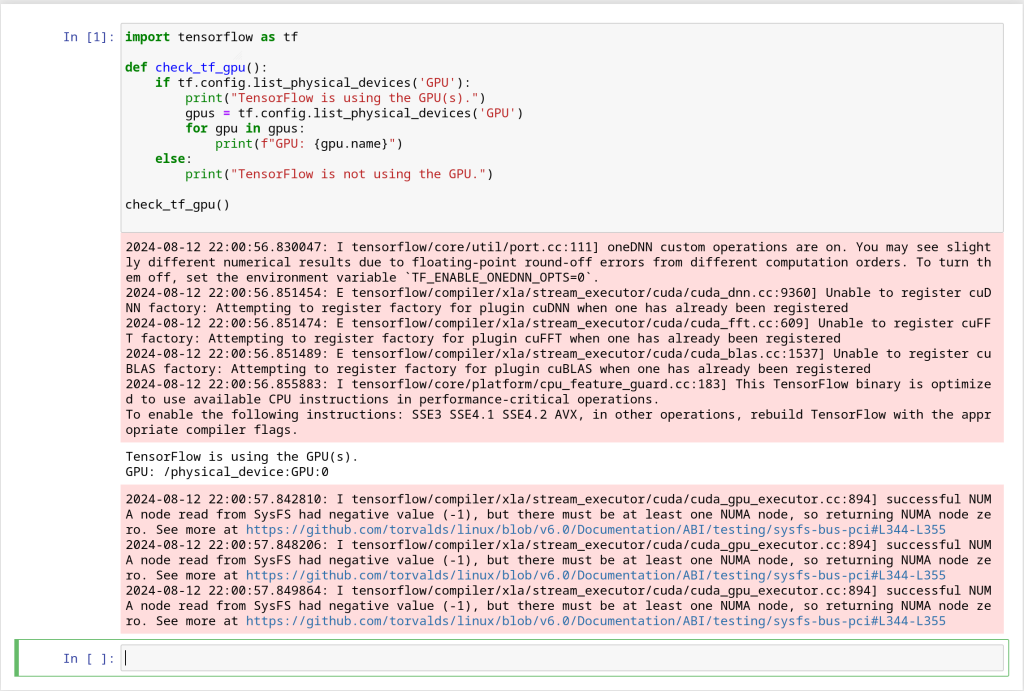
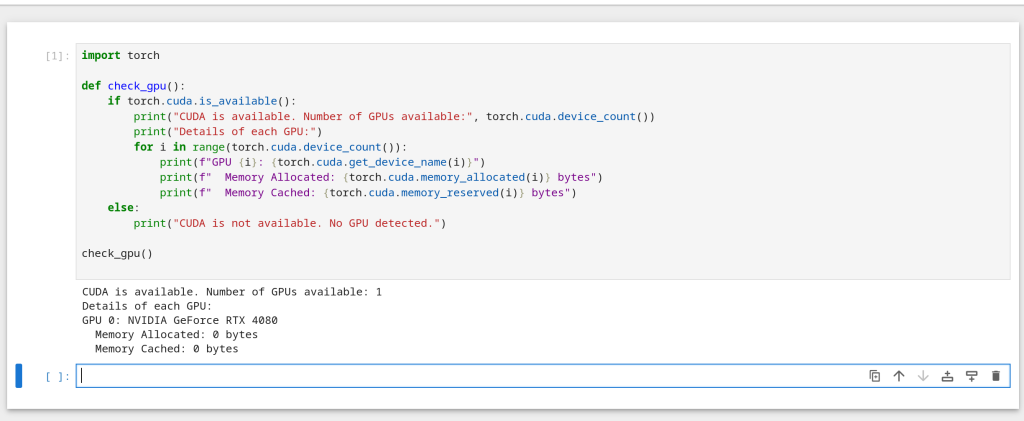
Simply desire to say your article is as surprising. The clearness for your put up is simply excellent and i can assume you are an expert on this subject. Well along with your permission allow me to seize your RSS feed to stay up to date with drawing close post. Thanks 1,000,000 and please continue the rewarding work.